FAST-EO: Enhancing Earth Observation with AI and machine learning techniques
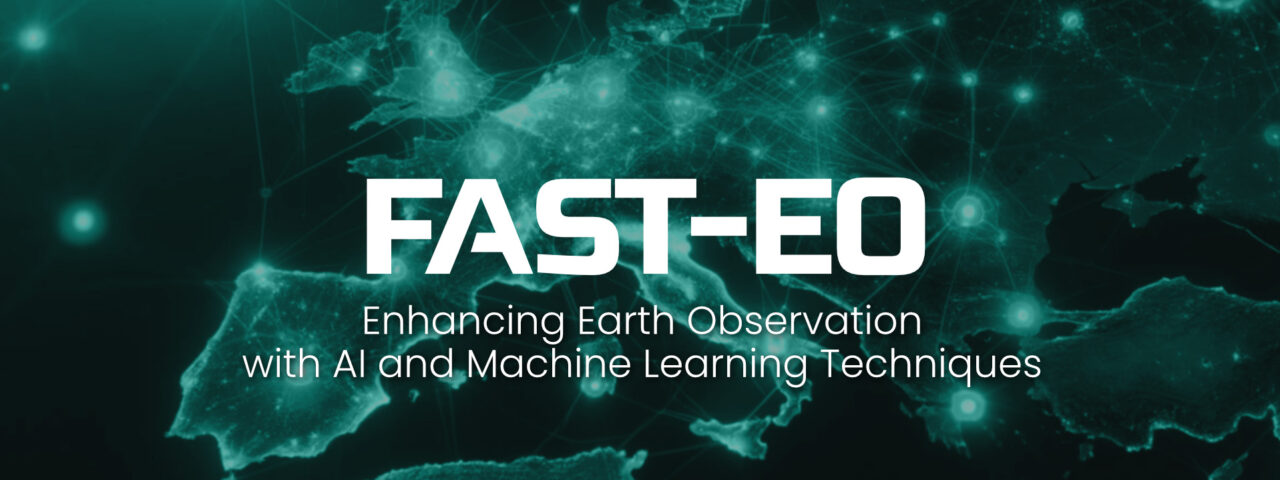
KP Labs is contributing to the FAST-EO project, a forward-thinking initiative launched in February under the auspices of the European Space Agency.
FAST-EO is the initiative aimed at merging Artificial Intelligence (AI) Foundation Models with Earth Observation Data. The project is a collaborative effort that brings together the expertise of the German Aerospace Center (DLR), Forschungszentrum Jülich, IBM Research, and KP Labs. Our collective effort is centered on utilizing satellite data, improving AI and machine learning techniques, and employing high-performance computing to address Earth Observation challenges. This approach facilitates innovative integration of AI with EO, aiming to advance environmental monitoring, climate research, and sustainable resource management.
The shift towards AI’s foundation models is predicated on training with vast amounts of unlabeled data. This approach enables seamless adaptation to various tasks and streamlines AI’s integration into fields like Earth Observation. It reduces the reliance on intensive data labeling and custom coding, enhances operational efficiency, and encourages innovation, particularly within hybrid-cloud environments.
In this project, machine learning techniques are leveraged to analyze extensive Earth Observation datasets, often unprocessed due to their sheer volume. By identifying anomalies and extending this analysis across different satellite-monitored regions, AI facilitates the discovery of similar patterns in small-scale areas, such as individual image pixels from Earth observations, enabling predictions and assessments of environmental phenomena on a broader scale.
FAST-EO sets its sights on several key areas of development:
Adapting AI models to meet the specific needs of Earth Observation and customizing these models to process and analyze the unique types of data encountered in EO applications. This includes developing methods to effectively handle and analyze satellite imagery and related data, requiring intricate algorithms to understand the complex nature of the information collected from space. The algorithms employed allow for the creation of further, even more effective models that are also linked with geographic data, thereby strengthening the role of this type of analysis in Earth observation.
Improving Multimodal Data Analysis by recognizing the diverse nature of EO data, which includes everything from images to text and sensor readings. FAST-EO is focused on enhancing AI models to not only work with a diverse array of Earth Observation data but also to merge these datasets with various geographic information from sources like aerial data, drone surveys, and subsoil data to extract more significant insights despite the complexities of integrating and interpreting such multifaceted data streams.
Making AI more affordable and deploying more accessible and advanced EO technologies. FAST-EO aims to find ways to lower the costs of technologies by focusing on developing more efficient AI training and deployment methods. Thanks to artificial intelligence, which will see connections between different areas and label them accordingly, previously unprocessed large datasets will become more accessible. This will, in turn, allow for better analysis of the further regions and the creation of algorithms necessary for further analysis. A small sample analyzed by AI will allow for the creation of models for a large dataset without the need for significant resources.
What’s KP Lab’s role in the project?
As KP Labs, we are playing a significant role in the FAST-EO project, especially in two use cases demonstrating the project’s practical impact. Our researchers focus on two topics:
Detecting Methane Leaks, where we develop the AI system designed to detect methane emissions using satellite data. This task involves analyzing spectral data and employing high-resolution images to identify methane leaks accurately. Why did we choose Methane?
While emissions of this gas don’t receive as much attention as, for example, CO2 statistics, Methane is equally, if not more, important to climate change; it traps 84 times more heat in the atmosphere than carbon dioxide, thus accounting for about one-quarter of the increase in temperature on our planet. Ensuring practical analysis of methane leaks will allow for more accurate predictions of its effects and, consequently, a reduction in its impact on the climate.


Photo credit: European Space Agency
Estimating Soil Properties, where we use the AI to determine soil properties from hyperspectral imagery. This approach promises to transform soil analysis, making it possible to assess soil conditions over large areas more efficiently and accurately, which could have significant implications for agriculture and land management. Soil analytics will not only allow farmers to plan their work better. It will also enable local governments and regions to effectively organize public policies related to agriculture and forestry and assess the necessity and justification for support in these areas. At the same time, it will allow local and regional policy representatives to stay in contact with beneficiaries of agricultural development grants to inform, support, and issue warnings in case of danger.
At KP Labs, we are on a quest to thoroughly investigate the fundamental principles of Foundation Models in the realm of Earth Observation. Our approach is meticulously crafted to balance rigorous research with hands-on application, intending to decode the intricate relationship between computational power and ethical norms. We’re committed to crafting a detailed and practical guide that will facilitate the smooth integration of these models into the EO field, ensuring that they meet the dynamic needs of our community today and in the times to come,” – says Jakub Nalepa, representing KP Labs in the FAST-EO Consortium.
What are the Broader Implications and Future Directions of FAST-EO?
By bringing together leading organizations to tackle these challenges, FAST-EO is paving the way for future Earth Observation and AI innovations. The project underscores the importance of collaborative research and open sharing of results to drive progress in this field. FAST-EO is committed to open-source principles, planning to provide its model weights, configurations for ensuring reproducibility, and datasets without any cost under an open and permissive license.
“This approach will guarantee full transparency and accessibility and encourage a user-friendly experience. Our efforts to make AI more adaptable to Earth Observation data and to enhance the processing of multimodal data will lead to more sophisticated and accessible EO technologies. Thanks to reducing the costs associated with these technologies, EO data will be more available to a broader range of users, from scientists and researchers to policymakers and environmental organizations,” – summarizes Jakub Nalepa.
More news
Stay informed with our latest blog posts.