Kameleon
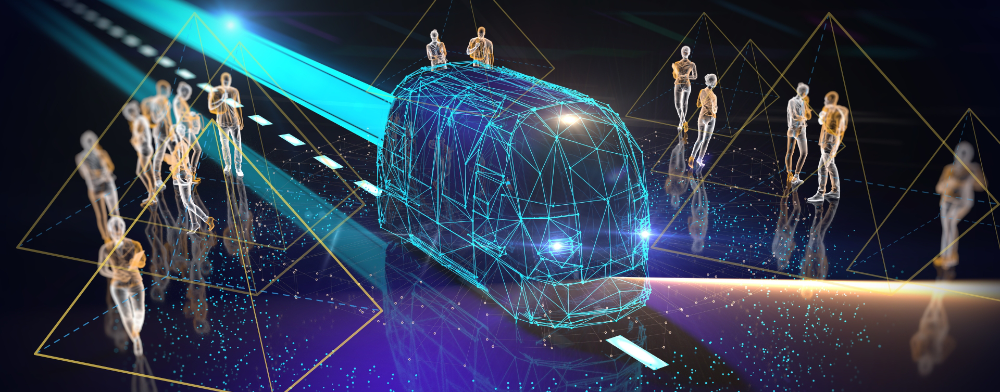
A Breakthrough Towards Autonomy
Kameleon automates the process of selecting sensors for a vehicle in the context of object detection in its surroundings, determines the optimal number of sensors, and evaluates the robustness of sensor configurations. The system is based on machine learning techniques utilizing deep neural networks with attention mechanisms. This enables the fusion of multimodal sensory data to create an accurate environmental map around the vehicle. It represents a step towards vehicle autonomy—from cars to satellites.
Distinctive features
Detection accuracy
Improving the accuracy of object detection in the vehicle’s surroundings.
Data fusion
Enhancing detection accuracy and robustness (e.g., against noise) in the vehicle's surroundings.
Optimization time
Reducing the time required to determine the vehicle’s sensor configuration.
System Advantages
- Optimization of sensor setup for vehicle perception, minimizing sensor costs while maximizing the robustness of sensor configurations against external conditions.
- Assessment of sensor (signal) relevance, optimizing the required number of sensors through:
- Data-driven algorithms (attention modules)
- Correlation with perception quality
- Redundancy management
- Evaluation of data fusion algorithm robustness against noisy and low-quality data through:
- A noise simulator with various distributions
- Quantitative, qualitative, and statistical assessment
The system was developed as part of the project:
"System for automatic optimization of vehicle sensor configuration, reliability assessment, and multimodal sensory data analysis using machine learning techniques (KAMELEON)" (Application No. WND-RPSL.01.02.00-24-0ACE/20-002).